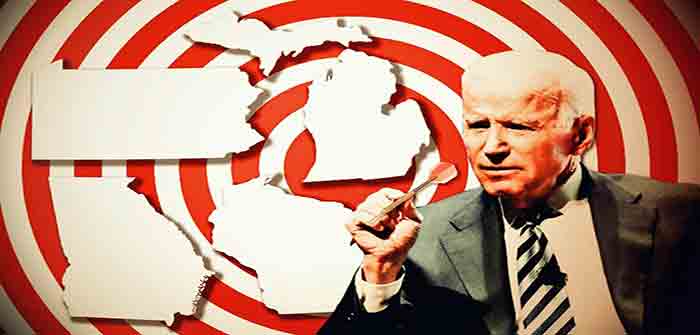
EXECUTIVE SUMMARY
We report a simple yet powerful statistical model of county-level voter behavior in the November 2020 presidential election using two main types of data:
- County-specific voting data from the five previous presidential elections.
- Selected demographic variables (race and education) plotting how different national voter groups voted differently in 2020 overall.
These two types of predictors allow us to explain over 95% of the variation in county-level votes, and therefore allow us identify which counties (and consequently, states) look substantially anomalous in the 2020 election.
The model provides substantial support for the allegation that the outcome of the election was affected by fraud in multiple states. Specifically, the model’s predictions match the reported results in all other states, i.e. states where no fraud has been alleged, but predicts Trump won majorities in five disputed states (AZ, GA, NV, PA and WI) and 49.68% of the vote in the sixth (MI).
In other words, the reported Biden margin of victory in at least five of the six contested states cannot be explained by any patterns in voter preference consistent with national demographic trends.
SUMMARY OF MAIN ARGUMENTS
1. Our model explains 96% of county-level variance in Trump’s two-party vote share with four demographic variables (non-college white, college-educated white, black and hispanic) and one historical variable (the average of county-level GOP two-party presidential vote share, 2004-2016). All five variables are highly significant. This reinforces the conclusion that the model is generally a very strong predictor of vote shares, and so deviations from it should be considered surprising.
2. Under conservative assumptions, regression analysis shows Trump ought to have won AZ, GA, NV, PA, WI.
[See the end of the article for the full table.]
3. Every one of the contested states shows a larger predicted vote share for Trump than what he actually received. This is surprising, because in any set of observations, random chance might expect some predictions to favor Biden, but none do. In Georgia and Arizona, the model does not predict a narrow race, but a decisive Trump victory; the size of the anomaly is (much) larger than the reported margin of victory.
4. The model also performs well in battleground states that have not been contested, and thus where the election was presumably clean. Every one of these is correctly predicted, including both battleground states that voted for Trump (e.g. Ohio, Florida) and those that voted for Biden (e.g. New Hampshire). Indeed, there are no states that Trump won which the model predicts should have been won by Biden. Meanwhile, the errors in the model are constructed to average to zero, so the model cannot favor one candidate over the other. Instead, it reveals the places where actual outcomes differ the most from our predictions.
5. The model is robust to alternative specifications of the regression formula and weighting.
6. The model places the burden of proof on fraud skeptics to explain why nearly all the states where fraud has been alleged, and only those states, have results inconsistent with statistical trends in the rest of the country.
7. Our model highlights the importance of a systematic comparison of all counties in the US when trying to understand whether the contested states are actually unusual. Simply picking isolated comparison cities, or one-off comparisons to past elections, is a very inferior way of doing the comparison. This model takes this base intuition (which is actually good), but greatly improves it by making the comparison systematic. The fact that the contested states are mostly predicted to have been won by Trump using simple but powerful demographic models further adds weight to the existing evidence that these outcomes may have been altered by fraud.
MAIN ANALYSIS
DATA
Our analysis used the following county-level datasets:
“total_results_CONDENSED.csv” [link]
“county_pres_2000_2016_source_MIT.csv” [MIT Election Lab]
“ACSST5Y2018.S1501_data_with_overlays_2020-11-16T170124.csv” (U.S. Census)
“cc-est2019-alldata.csv” (U.S. Census)
The demographic variables use US Census 2019 total population figures for non-hispanic white, black, and white hispanic to generate the white, black (“b”) and hispanic (“h”) categories, respectively. Working-class (“wwc”) and professional-class (“wpc”) whites were further distinguished using US Census educational attainment data (variables S1501_C01_031E, S1501_C01_033E).
County average historical GOP two-party vote share for presidential elections (“avg”) is an unweighted average of results for the 2004, 2008, 2012, and 2016 elections in the MIT dataset. Trump’s 2020 two-party vote share is derived from vote totals for 3106 counties in the lower 48 contiguous United States in “total_results_CONDENSED”.
THE MODEL…
Continue Reading